Corporate spending plans for artificial intelligence (AI) remain bullish, with investment expected to double by 2025 [1]. While companies remain optimistic, investors and regulators may be less so. As the initial hype fades, the pressure to deliver a return on investment grows, alongside demands for transparency and responsibility. One of the biggest—and perhaps least expected—challenges may be data.
Even as we generate vast amounts of data daily, the “all-you-can-eat buffet” for AI models is increasingly constrained. As the free-for-all ends, it will become clear which companies have built strong foundations and which have taken shortcuts.
Garbage in, garbage out: Cautionary tales from healthcare
AI has particularly excelled in the healthcare sector, transforming everything from clinical workflows to drug discovery [2]. However, this progress hinges on access to high-quality training data.
Training a model on limited, poor-quality or biased data can result in products that don’t work as hoped. Take IBM Watson Health, for example. After investing billions of U.S. dollars in a program to revolutionise cancer treatment through AI, IBM ultimately abandoned the project [3]. Among the issues was the system’s reliance on a limited dataset, which introduced bias and hindered its applicability to broader patient populations [4].
Access to larger datasets is helpful, but legal risks loom if data is obtained without proper consent. Google’s Project Nightingale raised alarms when it was revealed that the company accessed millions of Ascension Health’s records without patients’ prior knowledge, prompting an investigation by the U.S. Department of Health and Human Services [5]. Privacy regulations require companies to obtain consent for using patient data beyond treatment purposes, yet more than a quarter of companies examined had policies that were unclear or nonexistent.
Health-care companies’ consent policies on use of personal data for secondary purposes
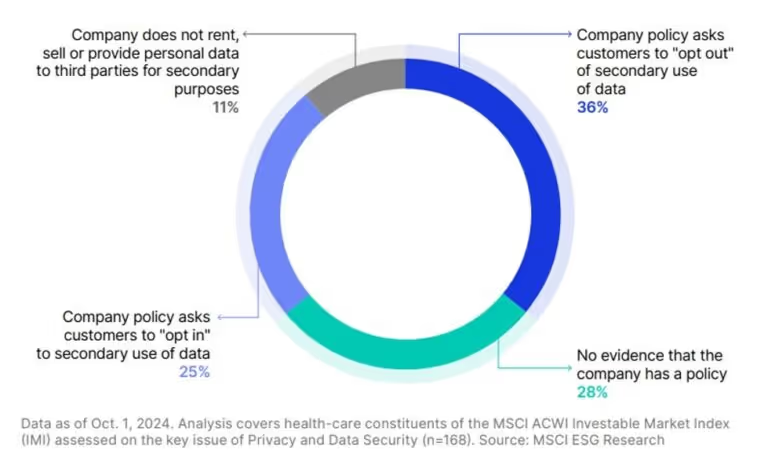
Matters of consent: Cutting off the data supply
Further regulatory constraints on legal access to data are becoming a reality, as major markets introduce AI-specific legislation on top of existing privacy laws. The EU AI Act takes effect this year, and California will require companies to disclose the datasets used to develop AI systems or services [6].
When MSCI reviewed seven leading AI developers as of October 2024, only five disclosed the types of data used to train their models [7]. All five relied on some combination of publicly available information, licensed data and user data. While this approach may sound reasonable, public data isn’t always legally approved for commercial purposes. Privacy or copyright rules can still apply.
Publicly available information has long been a major source for training AI models. While public data may appear abundant, it carries challenges. Not all public data is reliable, and historic biases can impact the quality of AI models.
Moreover, the amount of data available for AI models to use is shrinking. Between 2023 and 2024, there was a 25% reduction in the availability of high-quality data, as more website owners blocked web crawlers from accessing their content [8].
As the outlook for AI regulation evolves, so will the risk on companies and investors. Regulators will likely prioritise privacy, copyright and discrimination or bias as they work to manage this new technology. Addressing these issues proactively could distinguish genuine AI success from a hype-driven spending spree.
Missing the data on use of data: Understanding corporate policy
AI usage has spread far beyond early adopters. MSCI analysis shows that all the biggest consumer-facing companies globally are already integrating AI into products and operations [9]. Yet understanding these companies’ approaches to responsible or ethical AI use remains difficult.
As of October 2024, nearly half of these firms lacked a responsible-use-of-AI policy. Only one—Adobe—explicitly committed to respecting copyright laws and avoiding the use of publicly available online data, thanks to its library of licensed content [10].
This lack of clarity may frustrate investors trying to assess the opportunities and risks related to evolving regulations and data accessibility.
Is transparency on the menu in 2025?
The first wave of investments in generative AI was built on high hopes and expectations.
For the next wave of AI investments to take off, companies may need to prove that their foundations—specifically, the quality and legality of their data—are solid.
This article was adapted from MSCI’s Sustainability and Climate Trends to Watch 2025 report.
1 “New EY research finds AI investment is surging, with senior leaders seeing more positive ROI as hype continues to become reality,” EY, July 15, 2024.
2 Sectors refer to Global Industry Classification Standard (GICS®) sectors. GICS is the global industry classification standard jointly developed by MSCI and S&P Global Market Intelligence.
3 “2022 Annual Report,” IBM, Feb. 28, 2023.
4 Casey Ross and Ike Swetlitz, “IBM Pitched Its Watson Supercomputer as a Revolution in Cancer Care. It’s Nowhere Close.” STAT, Sept. 5, 2017.
5 Rob Copeland and Sarah E. Needleman, “Google’s ‘Project Nightingale’ Triggers Federal Inquiry,” Wall Street Journal, Nov. 12, 2019.
6 “EU AI Act: first regulation on artificial intelligence,” European Parliament, June 18, 2024. “AB-2013 Generative artificial intelligence: training data transparency,” California Legislative Information, Sept. 30, 2024.
7 Amazon.com Inc., Anthropic PBC, Alphabet Inc., Inflection AI Inc., Meta Platforms Inc., Microsoft Corp. and OpenAI. We focused on this group of companies as they have been identified as leaders by the U.S. government. “FACT SHEET: Biden-Harris Administration Secures Voluntary Commitments from Leading Artificial Intelligence Companies to Manage the Risks Posed by AI,” White House, July 21, 2023.
8 Shayne Longpre, Robert Mahari, Ariel Lee et al., “Consent in Crisis: The Rapid Decline of the AI Data Commons,” Data Provenance Initiative, July 2024.
9 Analysis based on disclosures from the 50 largest companies (in total) by market cap across the following sectors: communication services, consumer discretionary, consumer staples, financials, health care and information technology.
10 “Our approach to generative AI with Adobe Firefly,”Adobe.com, accessed Oct. 15, 2024.
<hr>
Disclaimer: The views and opinions expressed in this article are solely those of the author(s) and do not necessarily reflect the view or position of the Responsible Investment Association Australasia (RIAA). This article is intended as general information and should not be considered investment advice. It is recommended to seek appropriate professional advice before making any investment decisions.
About the contributors
About the speakers


Meggin Thwing Eastman
Managing Director, Global Head of Sustainable Finance Research London
-
MSCI
Meggin Thwing Eastman is Global Head of Sustainable Finance Research for MSCI. MSCI is one of the largest providers of ESG Ratings and sustainability and climate analytics to global institutional investors globally. Meggin and her team oversee development of new research solutions for sustainable finance regulation and a range of other sustainable investment and transition objectives. Meggin’s team also authors essential guidance and applied research to help institutional investors, corporates and other capital market participants meet their sustainability goals.
Meggin is a frequent speaker and media commentator on ESG and climate investing. Recent publications include ESG & Climate Trends to Watch (2024 and 2023). Meggin has worked in the sustainable investment field since joining the former KLD Research & Analytics in 1998 She holds a BA from Williams College and an MA from the University of California, Berkeley.
Corporate spending plans for artificial intelligence (AI) remain bullish, with investment expected to double by 2025 [1]. While companies remain optimistic, investors and regulators may be less so. As the initial hype fades, the pressure to deliver a return on investment grows, alongside demands for transparency and responsibility. One of the biggest—and perhaps least expected—challenges may be data.
Even as we generate vast amounts of data daily, the “all-you-can-eat buffet” for AI models is increasingly constrained. As the free-for-all ends, it will become clear which companies have built strong foundations and which have taken shortcuts.
Garbage in, garbage out: Cautionary tales from healthcare
AI has particularly excelled in the healthcare sector, transforming everything from clinical workflows to drug discovery [2]. However, this progress hinges on access to high-quality training data.
Training a model on limited, poor-quality or biased data can result in products that don’t work as hoped. Take IBM Watson Health, for example. After investing billions of U.S. dollars in a program to revolutionise cancer treatment through AI, IBM ultimately abandoned the project [3]. Among the issues was the system’s reliance on a limited dataset, which introduced bias and hindered its applicability to broader patient populations [4].
Access to larger datasets is helpful, but legal risks loom if data is obtained without proper consent. Google’s Project Nightingale raised alarms when it was revealed that the company accessed millions of Ascension Health’s records without patients’ prior knowledge, prompting an investigation by the U.S. Department of Health and Human Services [5]. Privacy regulations require companies to obtain consent for using patient data beyond treatment purposes, yet more than a quarter of companies examined had policies that were unclear or nonexistent.
Health-care companies’ consent policies on use of personal data for secondary purposes
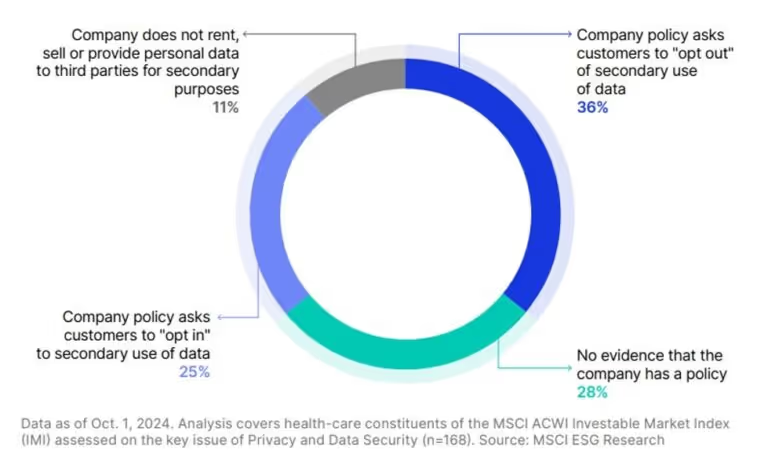
Matters of consent: Cutting off the data supply
Further regulatory constraints on legal access to data are becoming a reality, as major markets introduce AI-specific legislation on top of existing privacy laws. The EU AI Act takes effect this year, and California will require companies to disclose the datasets used to develop AI systems or services [6].
When MSCI reviewed seven leading AI developers as of October 2024, only five disclosed the types of data used to train their models [7]. All five relied on some combination of publicly available information, licensed data and user data. While this approach may sound reasonable, public data isn’t always legally approved for commercial purposes. Privacy or copyright rules can still apply.
Publicly available information has long been a major source for training AI models. While public data may appear abundant, it carries challenges. Not all public data is reliable, and historic biases can impact the quality of AI models.
Moreover, the amount of data available for AI models to use is shrinking. Between 2023 and 2024, there was a 25% reduction in the availability of high-quality data, as more website owners blocked web crawlers from accessing their content [8].
As the outlook for AI regulation evolves, so will the risk on companies and investors. Regulators will likely prioritise privacy, copyright and discrimination or bias as they work to manage this new technology. Addressing these issues proactively could distinguish genuine AI success from a hype-driven spending spree.
Missing the data on use of data: Understanding corporate policy
AI usage has spread far beyond early adopters. MSCI analysis shows that all the biggest consumer-facing companies globally are already integrating AI into products and operations [9]. Yet understanding these companies’ approaches to responsible or ethical AI use remains difficult.
As of October 2024, nearly half of these firms lacked a responsible-use-of-AI policy. Only one—Adobe—explicitly committed to respecting copyright laws and avoiding the use of publicly available online data, thanks to its library of licensed content [10].
This lack of clarity may frustrate investors trying to assess the opportunities and risks related to evolving regulations and data accessibility.
Is transparency on the menu in 2025?
The first wave of investments in generative AI was built on high hopes and expectations.
For the next wave of AI investments to take off, companies may need to prove that their foundations—specifically, the quality and legality of their data—are solid.
This article was adapted from MSCI’s Sustainability and Climate Trends to Watch 2025 report.
1 “New EY research finds AI investment is surging, with senior leaders seeing more positive ROI as hype continues to become reality,” EY, July 15, 2024.
2 Sectors refer to Global Industry Classification Standard (GICS®) sectors. GICS is the global industry classification standard jointly developed by MSCI and S&P Global Market Intelligence.
3 “2022 Annual Report,” IBM, Feb. 28, 2023.
4 Casey Ross and Ike Swetlitz, “IBM Pitched Its Watson Supercomputer as a Revolution in Cancer Care. It’s Nowhere Close.” STAT, Sept. 5, 2017.
5 Rob Copeland and Sarah E. Needleman, “Google’s ‘Project Nightingale’ Triggers Federal Inquiry,” Wall Street Journal, Nov. 12, 2019.
6 “EU AI Act: first regulation on artificial intelligence,” European Parliament, June 18, 2024. “AB-2013 Generative artificial intelligence: training data transparency,” California Legislative Information, Sept. 30, 2024.
7 Amazon.com Inc., Anthropic PBC, Alphabet Inc., Inflection AI Inc., Meta Platforms Inc., Microsoft Corp. and OpenAI. We focused on this group of companies as they have been identified as leaders by the U.S. government. “FACT SHEET: Biden-Harris Administration Secures Voluntary Commitments from Leading Artificial Intelligence Companies to Manage the Risks Posed by AI,” White House, July 21, 2023.
8 Shayne Longpre, Robert Mahari, Ariel Lee et al., “Consent in Crisis: The Rapid Decline of the AI Data Commons,” Data Provenance Initiative, July 2024.
9 Analysis based on disclosures from the 50 largest companies (in total) by market cap across the following sectors: communication services, consumer discretionary, consumer staples, financials, health care and information technology.
10 “Our approach to generative AI with Adobe Firefly,”Adobe.com, accessed Oct. 15, 2024.
<hr>
Disclaimer: The views and opinions expressed in this article are solely those of the author(s) and do not necessarily reflect the view or position of the Responsible Investment Association Australasia (RIAA). This article is intended as general information and should not be considered investment advice. It is recommended to seek appropriate professional advice before making any investment decisions.